Transformer Part 2 - Attention
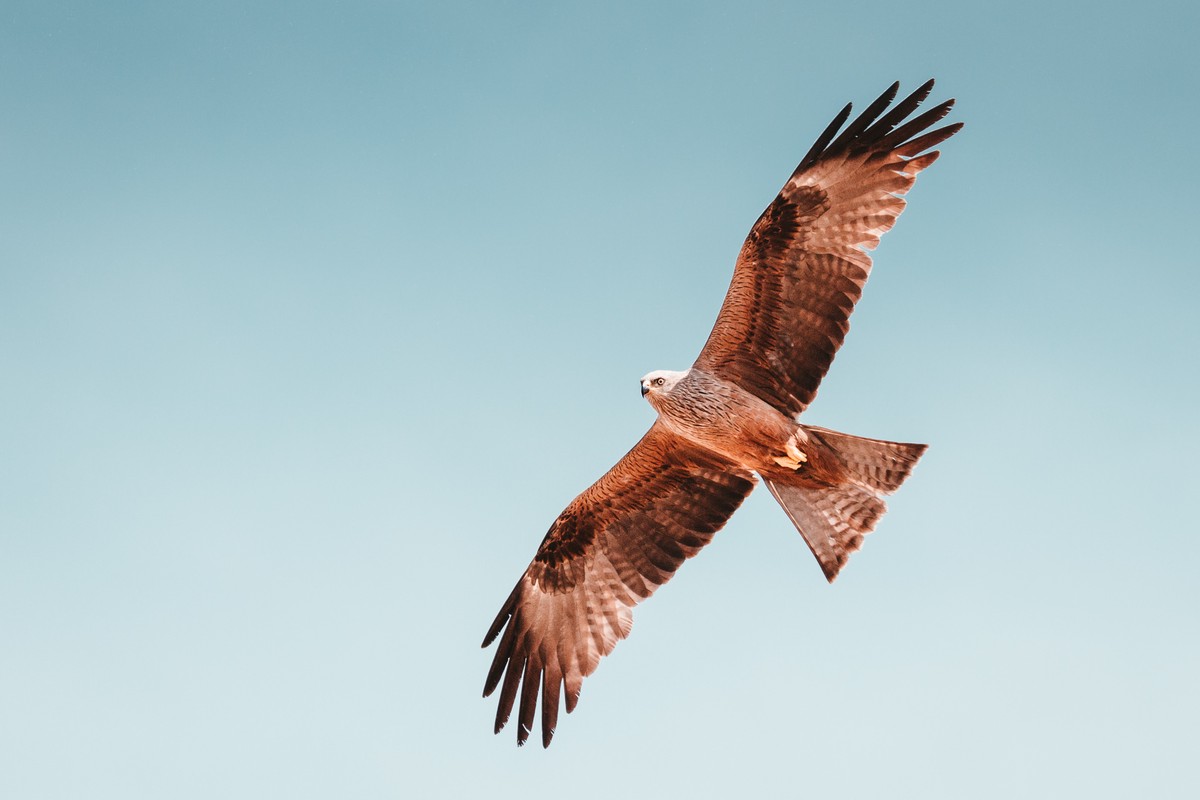
Attention Mechanism
Attention 的概念在 2014 年被 Bahdanau et al. [Paper 1] 所提出,解決了 encoder-decoder 架構的模型在 decoder 必須依賴一個固定向量長度的 context vector 的問題。實際上 attention mechanism 也符合人類在生活上的應用,例如:當你在閱讀一篇文章時,會從上下文的關鍵字詞來推論句子所以表達的意思,又或者像是在聆聽演講時,會捕捉講者的關鍵字,來了解講者所要描述的內容,這都是人類在注意力上的行為表現。
用比較簡單的講法來說, attention mechanism 可以幫助模型對輸入 sequence 的每個部分賦予不同的權重, 然後抽出更加關鍵的重要訊息,使模型可以做出更加準確的判斷。
複習一下在之前介紹的 Seq2Seq model 中,decoder 要預測在給定 context vector 與先前預測字詞 \({y_1, \cdots, y_{t-1}}\) 的條件下字詞 $y_{t}$ 的機率,所以 decoder 的定義是在有序的條件下所有預測字詞的聯合機率:
\[ \begin{align} p(\mathrm{y}) & = \prod_{t=1}^T p(y_t | \{y_1, \cdots, y_{t-1}\}, c) \tag 1 \\ \mathrm{y} & = (y_1, \cdots, y_T) \end{align} \]
在第 $t$ 時間,字詞 $y_t$ 的條件機率:
\[ \begin{align} p(y_t | \{y_1, \cdots, y_{t-1}\}, c) = g(y_{t-1}, s_t, c) \tag 2 \end{align} \]
當中 $g$ 唯一個 nonlinear function,可以為多層的架構,$s_t$ 為 hidden state,c 為 context vector。
而在 Attention model 中,作者將 decoder 預測下一個字詞的的條件機率重新定義為:
\[ \begin{align} p(y_i | \{y_1, \cdots, y_{i-1}\}, \mathrm{x}) = g(y_{i-1}, s_t, c_i) \tag 3 \end{align} \]
當中 $s_i$ 表示 RNN 在 $i$ 時間的 hiddent state。
\[ \begin{align} s_i = f\left(s_{i-1}, y_{i-1}, c_i\right) \tag 4 \end{align} \]
將式子 (3) 與 (2) 相比就可以發現,每一個預測字詞 $y_i$ 對於 context vector 的取得,由原本都是固定的 C 轉變成 每個字詞預測都會取得不同的 $C_i$。
Bahdanau Attention model 的架構如圖1:

Context vector $c_i$ 是取決於 sequence of annotations \((h_1, h_2, \cdots, h_{T_x})\) 的訊息,annotation $h_i$ 包含了在第 $i$ 步下, input sequence 輸入到 econder 的訊息。計算方法是透過序列權重加總 annotation $h_i$,公式如下:
\[ \begin{equation} c_i = \displaystyle\sum_{j=1}^{T_x}\alpha_{ij}h_j \tag5 \end{equation} \]
其中 $i$ 表示 decoder 在第 $i$ 個字詞,$j$ 表示 encoder 中第 $j$ 個詞。
$\alpha_{ij} $ 則稱之為 attention distribution,可以用來衡量 input sequence 中的每個文字對 output sequence 中的每個文字所帶來重要性的程度,計算方式如下 : \( \begin{align} \alpha_{ij} & = softmax(e_{ij}) \\ & = \frac{exp(e_{ij})}{\sum_{k=1}^{T_x}exp(e_{ik})} \tag6 \\ \end{align} \)
\[ e_{ij} = a(s_{i-1}, h_j) \tag7 \]
計算 attention score $e_{ij}$ 中 $a$ 表示為 alignment model (對齊模型),是衡量 input sequence 在位置 $j$ 與 output sequence 位置 $i$ 這兩者之間的關係。 這邊作者為了解決在計算上需要 $T_{x} \times T_{y}$ 的計算量,所以採用了 singlelayer multilayer perceptron 的方式來減少計算量,其計算公式: \( \begin{align} a(s_{i-1}, h_j) = v_a^Ttanh(W_aS_{i-1} + U_ah_j) \tag8 \end{align} \)
其中 $W_a \in R^{n\times n},U_a \in R^{n \times 2n},v_a \in R^n$ 都是 weight。
另外作者在此採用了 BiRNN(Bi-directional RNN) 的 forward 與 backward 的架構,由圖一可以得知
- Forward hidden state 為 \((\overrightarrow{h_1}, \cdots, \overrightarrow{h_{T_x}})\)
- Backward hidden state 為 \((\overleftarrow{h_1}, \cdots, \overleftarrow{h_{T_x}})\)
- Concatenate forward 與 backward 的 hidden state,所以 annotation $h_j$ 為 \(\left[\overrightarrow{h_j^T};\overleftarrow{h_j^T}\right]^T\)
這樣的方式更能理解句子所要表達的意思,並得到更好的預測結果。
例如以下兩個句子的比較:
- 我喜歡蘋果,因為它很好吃。
- 我喜歡蘋果,因為它很潮。
下圖為 Bahdanau Attention model 的解析可以與圖1對照理解,這樣更能了解圖一的結構:
需要注意的一點是在最一開始的 decoder hidden state $S_0$ 是採用 encoder 最後一層的 output

下圖為論文中英文翻譯成法語的 attention distribution:
在圖中 $[European \space Economic \space Area]$ 翻譯成$ [zone \space \acute{a}conomique \space europ\acute{e}enne] $ 的注意力分數上,模型成功地專注在對應的字詞上。

最後作者後續還有實驗了採用 LSTM 來替換 Vainlla RNN 進行實驗,詳細的公式都有列出在論文中,有興趣的可以看一下。
Attention Mechanism Family
Hard Attention & Soft Attention
Xu et al. [Paper 2] 對於圖像標題(caption)的生成研究中提出了 hard attention 與 soft attention 的方法,作者希望透過 attention mechanism 的方法能夠讓 caption 的生成從圖像中獲得更多有幫助的訊息。下圖為作者所提出的模型架構:

模型結構
- Encoder
在 encoder 端模型使用 CNN 來提取 low-level 的卷積層特徵,每一個特徵都對應圖像的一個區域
\[ a = \{a_1, \dots, a_L\}, a_i \in R^D \]
總共有 $L$ 個特徵,特徵向量維度為 $D$。
- Decoder
採用 LSTM 模型來生成字詞,而因應圖片的內容不同,所以標題的長度是不相同的,作者將標題 $y $ encoded 成一個 one-hot encoding 的方式來表示
\[ y = \{y_1, \dots, y_C\}, y_i \in R^K \]
K 為字詞的數量,C 為標題的長度。下圖為作者這本篇論文所採用的 LSTM 架構:
利用 affine transformation 的方式 \(T_{s, t} : R^s \rightarrow R^t\) 來表達 LSTM 的公式:
\[ \begin{pmatrix} i_t \\ f_t \\ o_t \\ g_t \end{pmatrix} = \begin{pmatrix} \sigma \\ \sigma \\ \sigma \\ tanh \end{pmatrix} T_{D+m+n, n} \begin{pmatrix} Ey_{t-1} \\ h_{t-1} \\ \hat{Z_t} \end{pmatrix} \tag1 \\ \]
\[ \begin{align} c_t & = f_t \odot c_{t-1} + i_t \odot g_t \tag2 \\ h_t & = o_t \odot tanh(c_t) \tag3 \end{align} \]
其中
- \(i_t\) : input gate
- \(f_t\) : forget gate
- \(o_t\) : ouput gate
- \(g_t\) : canaidate cell
- \(c_t\) : memory cell
- \(h_t\) : hidden state
- \(Ey_{t-1}\) 是詞 \(y_{t-1}\) 的 embedding vector,\(E \in R^{m \times k}\) 為 embedding matrix,m 為 embedding dimention
- \(\hat{Z} \in R^D\) 是 context vector,代表捕捉特定區域視覺訊息的上下文向量,與時間 $t$ 有關,所以是一個動態變化的量
特別注意的是作者在給定 memory state 與 hidden state 的初始值的計算方式使用了兩個獨立的多層感知器(MLP),其輸入是各個圖像區域特徵的平均,計算公式如下:
\[ \begin{align} c_0 = f_{init, c}( \frac{1}{L} \sum_{i}^L a_i) \\ h_0 = f_{init, h}( \frac{1}{L} \sum_{i}^L a_i) \end{align} \]
以及作者為了計算在 $t$ 時間下所關注的 context vector \(\hat{Z_t}\) 定義了 attention machansim $\phi$ 為在 $t$ 時間,對於每個區域 $i$ 計算出一個權重 \(\alpha_{ti}\) 來表示產生字詞 $y_t$ 需要關注哪個圖像區域 annotation vectors $a_i, i=1, \dots, L$ 的訊息。
權重 \(\alpha_i\) 的產生是透過輸入 annotation vector \(a_i\) 與前一個時間的 hidden state $h_{t-1}$ 經由 attention model $f_{att}$ 計算所產生。
\[ \begin{align} e_{ti} = f_{att}(a_i, h_{t-1}) \tag4 \\ \alpha_{ti} = \frac{exp(e_{ti})}{\sum_{k=1}^{L}exp{e_{tk}}} \tag5 \\ \hat{Z_t} = \phi(\{a_i\}, \{\alpha_{i}\}) \tag6 \end{align} \]
有了上述的資訊,在生成下一個 $t$ 時間的字詞機率可以定義為:
\[ p(y_t | a, y_1, y_2, \dots, y_{t-1}) \propto exp(L_o(Ey_{t-1} + L_hh_t + L_z\hat{Z_t})) \tag7 \]
其中 \(L_o \in R^{K \times m}, L_h \in R^{m \times n}, L_z \in R^{m \times D}\),m 與 n 分別為 embedding dimension 與 LSTM dimension。
對於函數 $\phi$ 作者提出了兩種 attention machansim,對應於將權重附加到圖像區域的兩個不同策略。根據上述的講解,搭配下圖為 Xu et al. [Paper 2] 的模型架構解析,更能了解整篇論文模型的細節:

Hard attention (Stochastic Hard Attention)
在 hard attention 中定義區域變數(location variables) $s_{t, i}$ 為在 t 時間下,模型決定要關注的圖像區域,用 one-hot 的方式來表示,要關注的區域 $i$ 為 1,否則為 0。
$s_{t, i}$ 被定為一個淺在變數(latent variables),並且以 multinoulli distriubtion 作為參數 $\alpha_{t, i}$ 的分佈,而 $\hat{Z_t}$ 則被視為一個隨機變數,公式如下:
\[ p(s_{t, i} = 1 | s_{j, t}, a) = \alpha_{t, i} \tag8 \]
\[ \hat{Z_t} = \sum_{i} s_{t, i}a_i \tag9 \]
定義新的 objective function $L_s$ 為 marginal log-likelihood $\text{log }p(y|a)$ 的下界(lower bound)
\[ \begin{align} L_s & = \sum_s p(s|a)\text{log }p(y|s,a) \\ & \leq \text{log } \sum_s p(s|a)p(y|s,a) \\ & = \text{log }p(y|a) \end{align} \]
在後續的 $L_s$ 推導求解的過程,作者利用了
- Monte Carlo 方法來估計梯度,利用 moving average 的方式來減小梯度的變異數
- 加入了 multinouilli distriubtion 的 entropy term $H[s]$
透過這兩個方法提升隨機算法的學習,作者在文中也提到,最終的公式其實等價於 Reinforce learing。作者在論文中有列出推導的公式,有興趣的可以直接參考論文。
Soft attention (Deterministic Soft Attention)
Soft attention 所關注的圖像區域並不像 hard attention 在特定時間只關注特定的區域,在 soft attention 中則是每一個區域都關注,只是關注的程度不同。透過對每個圖像區域 $a_{i}$ 與對應的 weight $\alpha_{t,i}$ ,$\hat{Z}_t$ 就可以直接對權重做加總求和,從 hard attention 轉換到 soft attention 的 context vector:
\[ \hat{Z_t} = \sum_{i} s_{t, i}a_i \implies \mathbb{E}{p(s_t|a)}[\hat{Z_t}] = \sum_{i=1}^L \alpha_{t,i}a_i \]
這計算方式將 weight vector $\alpha_i$ 參數化,讓公式是可微的,可以透過 backpropagation 做到 end-to-end 的學習。其方法是參考前面所介紹的 Bahdanau attention 而來。
作者在這邊提出三個理論:
- \(\mathbb{E}{p(s_t|a)}[h_t]\) 等同於透過 context vector \(\mathbb{E}{p(s_t|a)}[\hat{Z_t}]\) 使用 forward propagation 的方法計算 $h_t$
- Normalized weighted geometric mean approximation
- 根據公式(7)定義 \(n_t = L_o(Ey_{t-1} + L_hh_t + L_z\hat{Z_t})\)
所以 soft attention 在最後做文字的預測時作者定義了 softmax $k^{th}$ 的 normalized weighted geometric mean。
\[ \begin{align} NWGM[p(y_t=k|a)] & = \frac{\prod_i exp(n_{t,k,i})^{p(s_{t,i} = 1 | a)}}{\sum_j\prod_i exp(n_{t,j,i})^{p(s_{t,i} = 1 | a)}} \\ & = \frac{exp\left(\mathbb{E_{p(s_t) | a}[n_{t,k}]}\right)}{\sum_j exp\left(\mathbb{E_{p(s_t) | a}[n_{t,j}]}\right)} \end{align} \]
\[ \mathbb{E}[n_t] = L_o(Ey_{t-1} + L_h\mathbb{E}[h_t] + L_z\mathbb{E}[\hat{Z_t}]) \]
這邊的部分就是 soft attention 與 Bahdanau attention 的主要差異,在 Bahdanau attention 最後的 output 是透過 softmax 來取得下一次詞的機率,而作者在這邊採用了 NWGM 的方式。這邊並不是很清楚作者怎麼來證明這樣的論述,日後有理解出來或是有找到相關的參考再補上來。
最後來看看 soft attention 與 hard attention 的圖像視覺化結果,下圖是兩種 attention 對於圖像區域注意程度,可以看得出 hard attention 都會專注在很小的區域,而 soft attention 的注意力相對發散,這也是因為 soft 與 hard 在關注圖像區域上的一個是注意全部的圖像區域,一個是注意特定的區域。

Global Attention & Local Attention
Loung et al. [Paper 3] 在 2015 年所發表了 global / local attention 來提升 NMT 任務上的準確度,global attention 類似於 soft attention,而 local attention 則介於 hard 與 soft attention 的混合。
Global / local attention 相同之處:
- 採用的 target hidden state $h_t$ 是 stacking LSTM 最後一層的 output
- Context vector $c_t$ 都是將 $h_t$ 與 source-side $\bar{h_s}$ 作為 input 計算
- 結合 $c_t$ 與 $h_t$ 的訊息計算 \(\tilde{h_t} = tanh\left(W_c[c_t;h_t]\right)\),稱為 attentional vector
- 預測 $t$ 時間下的生成字詞的機率 \( p(y_t|y_{\text{<}t}, x) = softmax(W_s\tilde{h_t})\)
Global / local attention 不同之處:
- Context vector $c_t$ 的計算方式不同
- 採用 source side $\bar{h_s}$ 的數量不同
接下來分別介紹 global attention 與 local attention 當中的細節。
Global attention
作者將 alignment vector $a_t$ 定義是一個可變長度向量,所以在 global attention 中 $a_t$ 將全部時間的 source side 的資訊當作 input ,公式如下: \( \begin{align} a_t(s) &= align(h_t, \bar{h_s}) \tag 1 \\ &= \frac{exp(score(h_t,\bar{h_s}))}{\sum_{s'}exp\left(score(h_t,\bar{h_{s'}})\right)} \end{align} \) 在 score function 的部分,這邊定義了 content-based function,可以有以下三種形式:
\[ score(h_t, \bar{h_s}) = \begin{cases} h_{t}^T\bar{h_s} & \text{dot} \\ h_{t}^TW_a\bar{h_s} & \text{general} \\ v_a^Ttanh\left(W_a[h_t;\bar{h_s}]\right) & \text{concat} \end{cases} \]
其中 $W_a, v_a$ 都是透過訓練所得到的參數。
另外還有一種 local-based function,score只單存參考 target hidden state $h_t$ 的結果
\[ \begin{align} a_t = softmax(W_ah_t) && \text{location} \end{align} \]
下圖為 global attention 的模型架構:

Global attention 與 Bahdanau attention 對比,差異的地方如下:
- 在 encoder 與 decoder 都採用最後一層的 LSTM output 作為 hidden state
- 計算順序為 \(h_t \rightarrow a_t \rightarrow c_t \rightarrow \tilde{h_t}\),而 bahdanau attention 是 \(h_{t-1} \rightarrow a_t \rightarrow c_t \rightarrow h_t\)
Local attention
相較於 global attention 採用的所有 soucre side 的字詞,在計算上可能較為龐大,而且面對較長的句子可能無法翻譯正確的狀況,提出了 local attention,只專注關心一小部分的字詞來替代關注全部的字詞。
前面提到 local attention 是 hard 與 soft attention 的混合,選擇性地關注一個數量較小的上下文窗口,並且是可以微分的,減少了 soft attention 的計算量以及避免了 hard attention 不可微分的問題,更容易的訓練。
注意的重點:
- 在每個 $t$ 時間下生成 aligned position $p_t$
- Context vector $c_t$ 則是計算 $[p_t - D, p_t + D]$ 之間的 source hidden 做加權平均,$D$ 是可調的參數。如果所選擇的範圍超過句子本身的長度,則忽略掉多出來的部分,只考慮有存在的部分。
- Alignment vector $a_t \in R^{2D+1}$ 是固定的維度
作者針對模型提出了兩遍變形:
Monotonic aligment (local-m)
假設 source 與 target sequences 是單調對齊,就是指 source 與 target 長度相同: \( p_t = t \) Alignment vector $a_t$ 的計算就跟公式(1)一致。
Predictive alignment (local-p)
認為 source 與 target sequences 是並非單調對齊,就是長度不相同: \( p_t = S \cdot sigmoid\left( v_p^Ttanh(W_ph_t)\right) \) $W_p, v_p$ 都是可訓練的模型參數,$S$ 則表示 source sequence 的長度,$p_t \in [0, S]$。
Alignment vector $a_t$ 的計算採用的 Gaussian distribution 來賦予 alignment 的權重:
\[ a_t(s) = align(h_t,\bar{h_s})exp\left(-\frac{(s-p_t)^2}{2\sigma^2}\right) \]
$align(h_t,\bar{h_s})$ 與公式(1)一致,標準差設定為 $\sigma = \frac{D}{2}$,這是透過實驗所得來。
下圖為 local attention 的模型架構:

Local attention 可能的會遇到的問題:
- 當 encoder 的 input sequence 長度不長時,計算量並不會減少
- 當 Aligned position $p_t$ 不準確時,會直接影響到 local attention 的準確度
Input-feeding Approach
作者認為在 global 與 local attention 的方法中,模型的注意力機制是獨立的,但是在整個翻譯的過程中,必須要去了解哪些資訊已經被翻譯了,所以在預測下一個翻譯字詞時,應該結合過去 attentional vectors $\tilde{h_t}$ 的資訊,也就是說在 deocder 這邊多考慮了 alignment model 的結果,如下圖所示:

來看看論文中的針對各個模型在 WMT'14 英文翻譯成德文資料集的訓練結果:
- 這邊的實驗限制了字詞的數量,只取在資料集中最常出現的 50k 字詞當作 corpos
- 如果出現字詞沒有在 corpos 中,則用 <unk> 來取代

Global attention 與 local attention 都有自己的優勢,如果說要選用哪個方式來當作模型,認為因應不同的任務可能表現都會有所差異,所以建議兩種都實驗看看結果來比較優劣,而在實際上大多數採用的都是以 Global attention 為主。
底下列出上面各篇論文所提到的 Attention score function:
Name | Attention score function |
---|---|
Dot-product | $score(s_t, h_i) = S_t^Th_i$ |
General | $score(s_t, h_i) = S_t^TW_ah_i$ |
Additive | $score(s_t, hi) = v^Ttanh(WS{t-1} + Uh_i) $ |
Scaled Dot-product | $score(s_t, h_i) = \frac{S_t^Th_i}{\sqrt{d}}$ |
Loocation | $a_{t} = softmax(W_aS_t)$ |
總結來說:
Attention 的目的就是要實現的就是在 decoder 的不同時刻可以關注不同的圖像區域或是句子中的文字,進而可以生成更合理的詞或是結果。
Refenece
Paper:
- Dzmitry Bahdanau, KyungHyun Cho Yoshua Bengio, NEURAL MACHINE TRANSLATION BY JOINTLY LEARNING TO ALIGN AND TRANSLATE(2015)
- Kelvin Xu, Jimmy Ba, Ryan Kiros, Kyunghyun Cho, Aaron Courville, Ruslan Salakhudinov, Rich Zemel, and Yoshua Bengio, Show, Attend and Tell: Neural Image Caption Generation with Visual Attention(2015)
- Thang Luong, Hieu Pham, Christopher D. Manning, Effective Approaches to Attention-based Neural Machine Translation(2015)
- Sneha Chaudhari, Gungor Polatkan , Rohan Ramanath , Varun Mithal, An Attentive Survey of Attention Models(2019)
Illustrate:
- https://zhuanlan.zhihu.com/p/37601161h
- https://zhuanlan.zhihu.com/p/31547842
- https://blog.floydhub.com/attention-mechanism/#bahdanau-atth
- https://web.stanford.edu/class/cs224n/slides/cs224n-2019-lecture08-nmt.pdf
- https://www.cnblogs.com/Determined22/p/6914926.html
- https://jhui.github.io/2017/03/15/Soft-and-hard-attention/
- http://download.mpi-inf.mpg.de/d2/mmalinow-slides/attention_networks.pdf
- https://www.jiqizhixin.com/articles/2018-06-11-16
- https://medium.com/@joealato/attention-in-nlp-734c6fa9d983
- https://towardsdatascience.com/attn-illustrated-attention-5ec4ad276ee3
- https://lilianweng.github.io/lil-log/2018/06/24/attention-attention.html#a-family-of-attention-mechanisms
- https://zhuanlan.zhihu.com/p/80692530
Tutorial:
- Neural Machine Translation (seq2seq) Tutorial
- https://www.tensorflow.org/tutorials/text/transformerG
- Guide annotating the paper with PyTorch implementation
Visualization: